AI A/B Testing: How AI-Driven Optimization is Different
When it comes to experimentation, it’s all about the ability to prove hypotheses, improve experiences, and discover insights that contribute to business impact.
A/B testing is a common experimentation and conversion rate optimization tactic. But, because A/B testing is a highly involved task with a limited scope, organizations need help to maximize its impact.
By removing the complexity of building, deploying, optimizing, and analyzing A/B tests, AI empowers organizations to meet their goals with more velocity.
What is A/B Testing?
Concerning customer experience, A/B testing is a way to compare two variations of a digital touchpoint to see which performs better.
Let’s say you want to increase conversions on your homepage.
A basic example of A/B testing would be splitting website traffic 50/50 and showing two groups a slightly different homepage experience to see which converts more. For example, changing the color of a call-to-action (CTA) button in the HERO of your website from white to red.
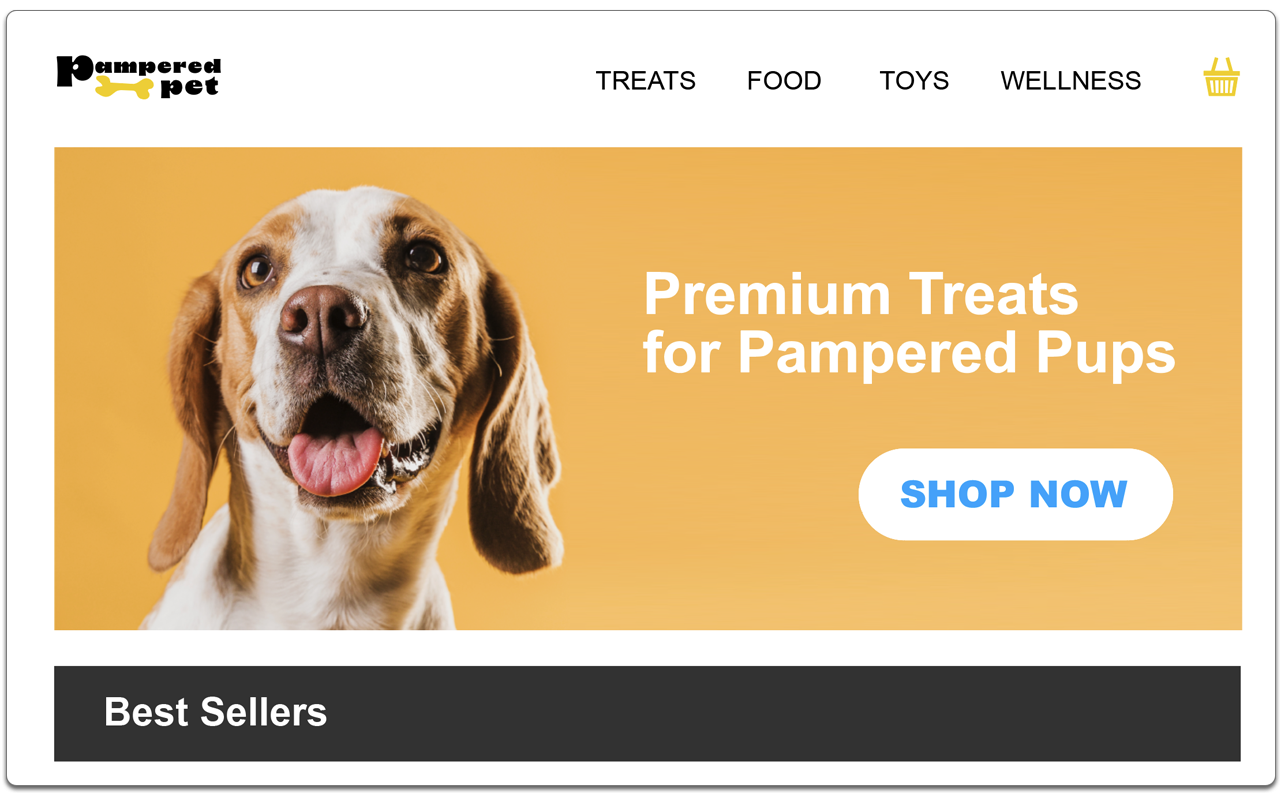
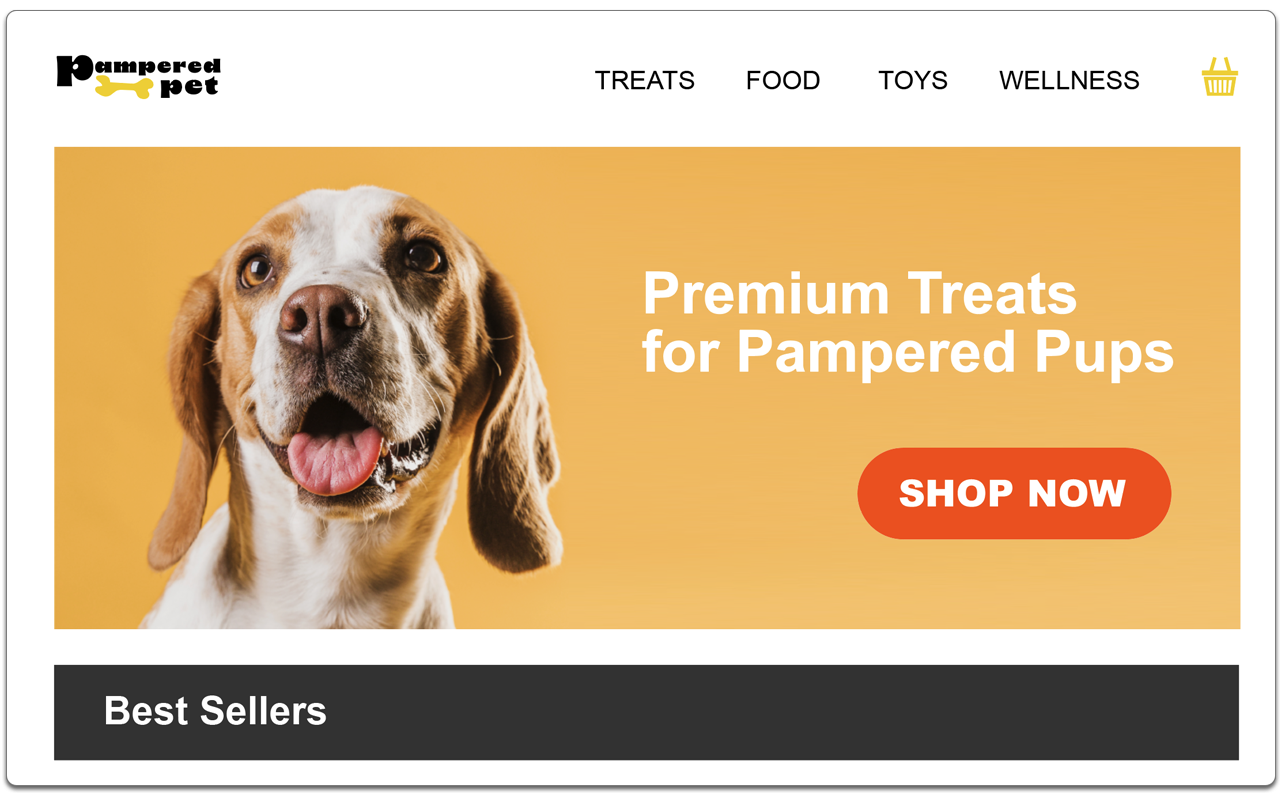
After generating enough data (sessions), an experimenter can see which variation of the CTA encourages more conversions.
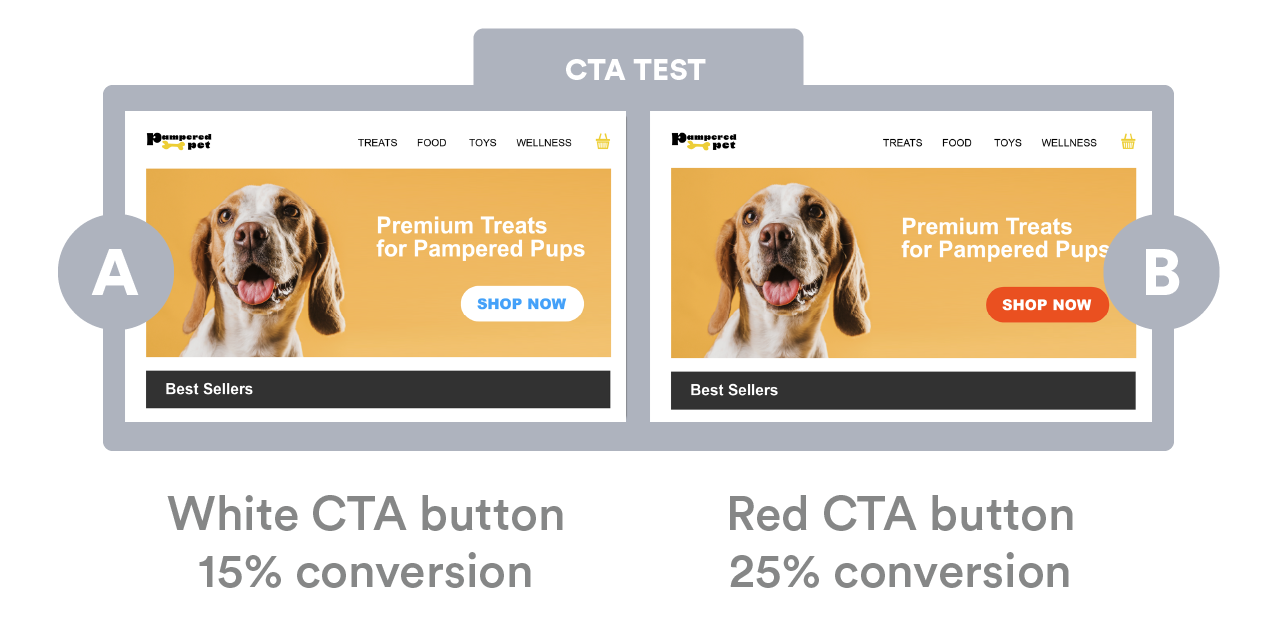
This example shows that the red CTA button generated 10% more conversions than the white version. Because the red CTA was the “winning” version, the next step would be to implement the change and show 100% of traffic the red CTA.
Limitations of A/B Testing
A/B tests are straightforward, which is why many marketing and product teams gravitate toward them. But they aren’t very efficient:
-
Depth: Testing A versus B is helpful for isolated changes, like the color of the CTA button. It’s clear which version performs better, and you can quickly implement the change. But, often, it’s not just the color of a CTA button that makes an impact. Significant changes happen due to many smaller changes across several pages and touchpoints. In most cases, you need to see more than just A versus B. A more insightful experiment would be A vs. B vs. C vs. D vs. E vs. F and beyond, or even combinations of changes like A with B and C vs. A with D and F.
-
Velocity: While websites that generate high volumes of traffic may be able to enjoy truncated testing periods, most organizations don’t have that luxury. Instead, A/B tests may need to run for months before reaching statistical significance. And if you see a flaw in the experience or an improvement that may have a better impact, with an A/B test, you cannot start and stop the test to course correct. You would need to start back at square one and let your new hypotheses run its entire course.
-
Lack of personalization: A/B tests are often called split tests because you’re doing just that: splitting traffic 50/50 for insights. The problem with 50/50 testing is that when an experience goes live, 100% of your users will receive the same version of an experience. And it’s hard to know how the 50/50 split will truly perform against the entirety of your audience, especially within select segments of your audience. For example, did your split test consider frequent shoppers versus first-time visitors? True personalization requires more testing, with deeper analysis before programmatically targeting rules for an experience.
-
Rigid findings: While A/B testing might be straightforward, the foundational statistics of A/B testing are rigid. A/B testing leverages Frequentist statistics, which draws conclusions from sample data by emphasizing the frequency or proportion of the data. In layman’s terms, it’s like saying, “Boom, we hit our mark. From this day forward, this is the answer.” The problem is users adjust their behaviors quickly, and the answer to your problems may change just as quickly. Without a more agile approach to experimentation, once the findings from your A/B test become invalidated, you’re back at square one.
Running multiple tests in the same funnel or on the same page is poor form as they can substantially affect each other. For that reason, and the bevy of bottlenecks outlined above, most brands can only run a handful of tests per year. And when you need to optimize several touchpoints across checkout pages, display ads, landing pages, email marketing - pretty much anywhere that copy, images, or placement - finding business impact from A/B testing starts to feel like an impossible task.
Still, experimentation is essential for risk mitigation and data-driven decision-making. Luckily, AI disruption has evolved what’s possible with A/B testing.
AI-led Optimization
Simply put, experimentation with AI enables brands to do more, better and faster.
AI allows marketers to try vastly more ideas in the same time frame as an A/B test and test across an entire funnel instead of a single page. AI also makes it easy to create dynamically updating experiences with unlimited versions while personalizing experiences based on your defined audiences.
-
Continuous optimization: Your experiences can “self-optimize” to concurrently target audiences, segments, or general audiences across multiple touchpoints. Unlike A/B testing, AI iterates on running experiments in real-time: removing poor-performing ideas and adding or removing new variants without starting and stopping an experiment. For example, suppose your top-performing combination increases orders but decreases units or items per order. In that case, AI can automatically add a variant that makes it easier to increase cart items without running a separate test.
-
Scale: A/B purists often complain about not being able to run enough good experiments. From ideating to deploying to analyzing and optimizing, A/B testing from start to finish is a lengthy process. Adding AI into the mix alleviates the challenges that cost your organization time. AI learns what’s working and what’s not and automatically recommends the next best UX actions. AI also helps build projects, from code and copy to imagery, making it possible to add new variants to experiments faster. According to one of our partners, “Evolv AI enabled us to run 6 years worth of experimentation in 3 months.”
-
Integrates: Your data ecosystem is critical in today’s digitally connected world. Adding insights for additional data sources into your manual A/B testing program increases the complexity of analysis. However, this process is automated with AI and handles the calculations for you. Data from multiple sources creates a more nuanced picture and, in the end, an actionable outcome.
Manually developing and deploying A/B tests can take developers months to build, especially when upper management calls for new features or functionalities at the last minute. With AI, you can use automation to create experiments on the fly while testing more rapidly and with less risk, helping to mature an experimentation program at scale.
Building Multivariate Tests with AI
Before you start testing, it’s important to understand what metric you’re trying to maximize. Common KPIs to experiment against are improvements in:
-
Average order value (AOV)
-
Units per order (UPO)
-
Sign-ups
-
Subscriptions
-
Checkouts
-
Bookings
-
Lifetime Value to Customer Acquisition Cost ratio
These KPIs are often directly correlated to a particular customer journey. For example, the KPI “checkouts” is connected to a checkout flow, which might include a product landing page, product detail page, checkout page, and a thank you page representing a completed conversion.
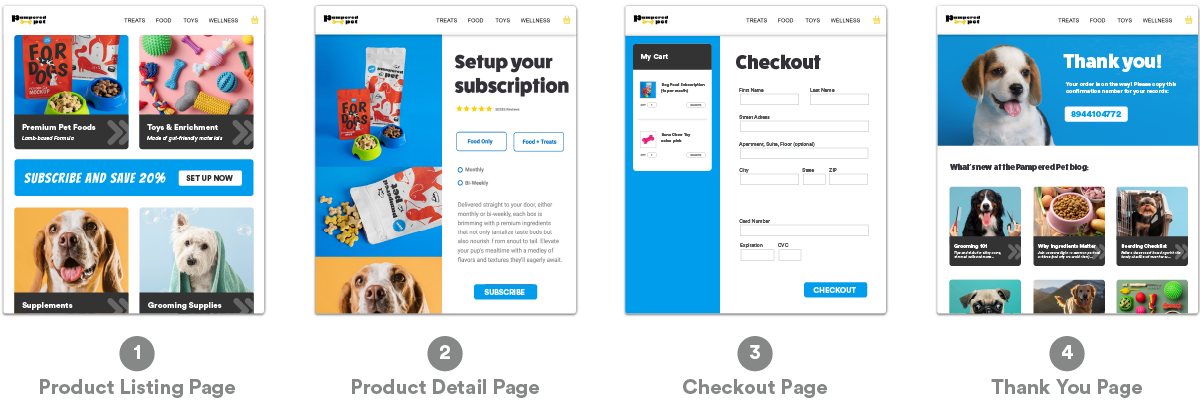
When optimizing for a KPI like “checkout completion,” it’s usually best practice to select the touchpoint of the flow with the highest value or the most likely friction point. For example, the checkout page may be the most essential because users have already made a choice to purchase - now you have to get them to complete the transaction.
So, let’s build a hypothesis that can improve the checkout page.
Your hypothesis can either be rooted in data or instinct. For example: “I don’t think our users know what’s in their cart at the checkout page. To increase performance, we should add cart details to the full checkout flow, and then more users will check out.”
You can also let Evolv AI’s recommendation engine provide suggestions for experiments that are likely to convert.
Here are some additional questions you can ask to help form your hypotheses:
-
What personal information is needed and why? Can I remove any questions for brevity? Should I add more for security?
-
Are the fields in which I ask for personal information ordered logically?
-
Is there more drop-off during big purchases than small? How can we gain the trust of consumers before asking for personal information?
-
Are cart items readily displayed?
-
Are you using the full width of the viewport on mobile?
-
Is there more than one CTA on the desktop version of the experience?
-
Are there actions needed besides simply reviewing your order and continuing?
-
How much information is visible? Should you collapse some or all of the order details?
-
Did you apply a promotion option on the PDP, and can you see it from the cart?
Elements on the checkout page to test in response:
-
Checkout button color
-
Checkout button placement
-
Add trust symbols
-
Change messaging for return visitors
-
Add social media sign-up options
-
Offer a guest checkout
-
Personalize cart
-
Update messaging to be clear and concise
-
Remove multiple CTAs
-
Highlighting free and/or fast shipping
-
Highlighting free and/or easy returns
-
Adding bundled products or a “you may also like” carousel with a 1-click add-to-cart
-
Show promotions clearly
You can use Evolv AI's library of UX recommendations to jump-start your experimentation program, and continuous optimization will ensure the best version of your experiences is served to the right audience and at the right time.
And, you don’t need to worry about the tactical work of developing the different versions of assets for your experiments either. With Generative AI, communicate your prompt to Evolv AI’s assistant, Cali, and generate images, text, and code for easy variant generation under the same roof as your experimentation platform.
Getting started with AI-led Experimentation
Machine learning algorithms sound like a complex undertaking – and they are for our developers– but for you, it’s a simple integration and a no/code-low/code experience.
All that’s required to get started with Evolv AI is a simple snippet in the head of your site.
-
Average order value (AOV)
-
Units per order (UPO)
-
Sign-ups
-
Subscriptions
-
Checkouts
-
Bookings
-
Lifetime Value to Customer Acquisition Cost ratio
These KPIs are often directly correlated to a particular customer journey. For example, the KPI “checkouts” is connected to a checkout flow, which might include a product landing page, product detail page, checkout page, and a thank you page representing a completed conversion.
Want to learn more about Evolv AI– the first AI-led experience optimization platform that recommends, builds, deploys, and optimizes customer experiences that impact customer behavior and drive business outcomes? Connect with us.